What's the Difference Between Big Data and Data Mining?
- Atul 1
- Sep 22, 2023
- 5 min read
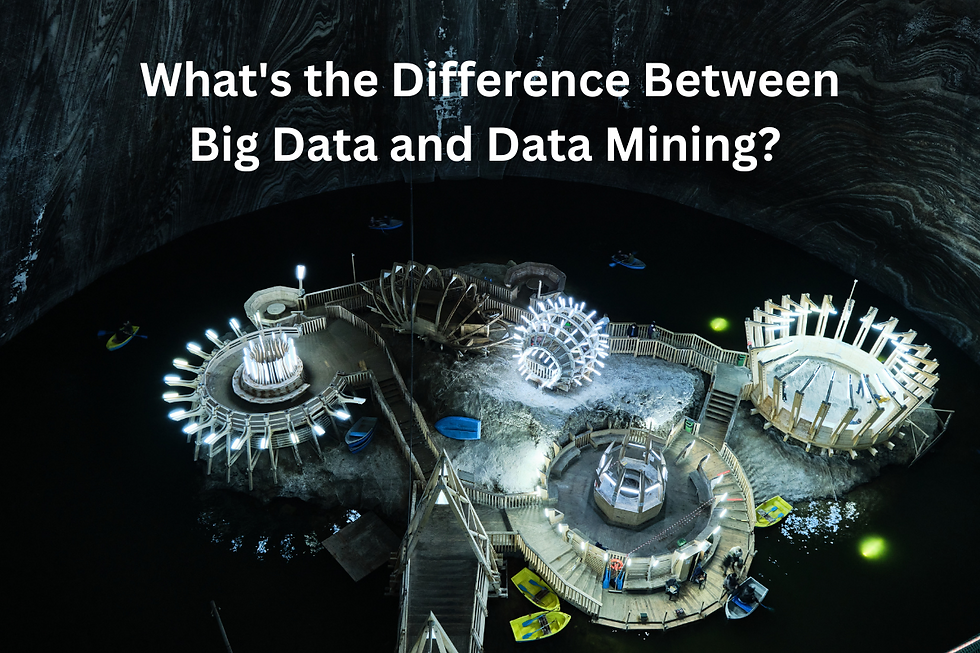
Introduction to Big Data and Data Mining
In today's digital age, data plays a crucial role in decision making processes for businesses and organizations. We will explore the differences between big data and data mining. With the advancements in technology, the amount of data being generated has increased exponentially, giving rise to the concepts of big data and data mining. Many people often use these terms interchangeably, but they are actually two distinct concepts with different purposes.
Understanding Big Data:
Big data refers to large and complex datasets that cannot be processed using traditional methods. It is characterized by its volume, velocity, and variety. Volume refers to the massive amount of data being generated from various sources such as social media platforms, online transactions, sensors, etc. Velocity refers to the speed at which this data is being generated and needs to be analyzed in real time. Lastly, variety refers to the different types of data such as structured, unstructured, and semistructured.
What is Data Mining?
Data mining is a process of extracting useful insights and patterns from raw data. It involves using techniques such as machine learning, statistics, and artificial intelligence to analyze large datasets. The goal of data mining is to discover hidden patterns or relationships that can help businesses make informed decisions.
Key Differences Between Big Data and Data Mining:
1) Purpose:
The primary purpose of big data is to store and manage large amounts of information effectively. On the other hand, data mining focuses on extracting meaningful insights from this vast amount of information.
2) Data Volume:
As mentioned earlier, big data deals with a massive volume of information that traditional processing methods cannot handle. In comparison, dealing with structured data sets for analysis purposes only requires a smaller dataset.
Definition of Big Data
To start off, let’s define what Big Data is. Simply put, it refers to a large volume of structured or unstructured data that is difficult to process using traditional database management tools. This data comes from various sources such as social media posts, online transactions, sensor readings, and more. But what sets Big Data apart from regular data is not just its size but also its complexity and speed at which it is generated.
Now you may be thinking, how much data does it take to qualify as Big Data? Well, there’s no definite answer to this question as the amount of data that makes up Big Data keeps increasing every year. However, according to IBM’s 4 V's model – Volume, Variety, Velocity, and Veracity – if the data meets these criteria then it can be considered as Big Data. Let’s break down these characteristics:
1) Volume: As mentioned earlier, the size of the data plays a crucial role in determining whether it falls under the category of Big Data or not.
2) Variety: The type and format of data also contribute to its complexity. With Big Data, you can have structured (e.g., databases) and unstructured (e.g., videos) data coming from various sources.
3) Velocity: The speed at which new data is generated determines how quickly your system needs to process it.
Definition of Data Mining
Firstly, what exactly is data mining? In simple terms, it is the process of analyzing large sets of data to uncover hidden patterns and insights. These patterns may not be immediately apparent or identifiable without the use of specialized tools and techniques.
On the other hand, big data refers to the sheer volume of digital information being generated by various sources such as social media, online transactions, sensors, etc. This enormous amount of data is continuously growing at an exponential rate and provides a goldmine for businesses looking to gain insights into consumer behavior or market trends.
Now that we have a basic understanding of both concepts let's dive deeper into their key differences:
1. Purpose
The main purpose of data mining is to extract valuable insights or knowledge from existing datasets. It involves identifying patterns and relationships within the raw data that can be used for future decision making. On the other hand, big data's primary purpose is to store and manage massive amounts of information effectively.
2. Methodology
Data mining requires advanced statistical algorithms and machine learning techniques to analyze data sets and find meaningful patterns. These methods involve identifying correlations between variables, creating models for prediction or classification, and detecting anomalies in the data set.
Characteristics of Big Data
Let's get started by defining what exactly we mean by big data and data mining. Big data refers to extremely large datasets that are too complex to be managed or processed by traditional database systems. These datasets can range from a few terabytes to even petabytes of structured, unstructured, and semistructured data.
The first major difference between big data and data mining lies in their scope or size of datasets. While both deal with large amounts of data, big data typically refers to datasets that are so massive that traditional software tools cannot effectively handle them. Data mining, on the other hand, deals with subsets of these massive datasets that have been specifically selected for analysis based on certain criteria.
Another crucial aspect that sets big data apart from data mining is the importance of technology in managing and processing these datasets. Big data requires specialized hardware and software solutions to store, manage, process, and analyze it effectively. This is because traditional database systems often struggle with handling such massive volumes of information.
Characteristics of Data Mining
Big data refers to the vast amount of structured and unstructured data that organizations can collect and analyze in order to gain insights and make informed decisions. This includes everything from customer transactions to social media interactions to sensor data from machines.
On the other hand, data mining is a process of extracting useful information or patterns from big data through various techniques such as machine learning. In simple terms, it is like searching for a needle in a haystack – finding valuable insights within large volumes of complex and unstructured information.
One of the key characteristics of data mining is its ability to discover hidden patterns or relationships within big data sets that may not be obvious at first glance. This allows organizations to make predictions and identify trends that can inform business decisions.
Another important characteristic of data mining is its ability to handle both structured and unstructured data. Unlike traditional methods of analysis that only work with structured information (e.g., databases), modern data mining techniques are able to handle unstructured data such as text, images, videos, and more.
Applications and Uses for Big Data
Now that we have established the difference between these two terms let's delve into how big data is utilized in various industries.
Business Intelligence:
Big data has revolutionized the way businesses operate. With the help of advanced analytics tools, companies can now gather vast amounts of customer information from different sources such as social media, website traffic, and purchase history. This information can then be processed to gain valuable insights into customer behavior and preferences.
Healthcare:
Big Data plays a crucial role in healthcare by allowing healthcare providers to analyze patient records on a large scale. By combining medical records with genetic information, health patterns can be identified that determine which treatments work best for certain conditions or diseases.
Comments