Pandemic Forecasting: Making predictions about the future using Machine Learning
- Atul 1
- Sep 18, 2023
- 6 min read
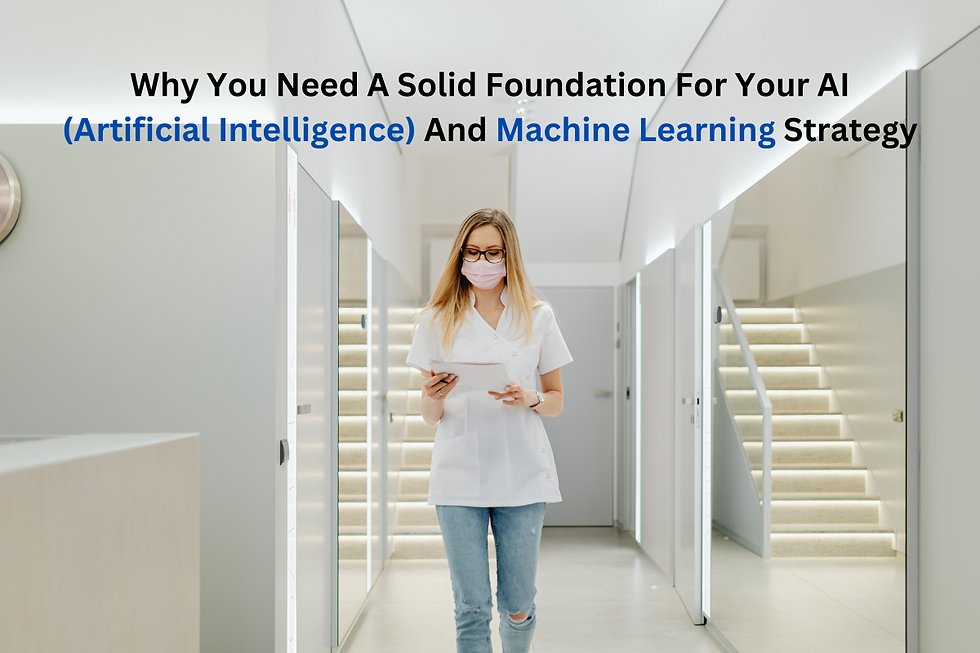
Overview of Pandemic Forecasting using Machine Learning
Pandemic forecasting is a field that has seen great advances in predicting pandemics and understanding their path of progression. In this blog post, we will take an overview of how pandemic forecasting works, the importance of machine learning in making predictions about the future, data collection and modeling accuracy, as well as predictions related to future trends.
Pandemic forecasting is a key tool for healthcare professionals to understand how a virus or disease may spread based on historical data. Predictive models powered by machine learning can sift through vast amounts of past data and identify patterns that can be used to predict future trends, as well as areas where interventions might be timely or effective.
Data collection is crucial when it comes to using predictive models and machine learning algorithms for pandemic forecasting. Data must be up to date and accurate in order for these tools to work effectively. To ensure accuracy, healthcare organizations should focus on collecting data from multiple sources such as public health agencies, hospitals, research studies, and surveys about individual risk factors like smoking habits or pre-existing conditions.
Understanding the Data & Models Used in Machine Learning
Making predictions about the future amid a pandemic can be difficult – but with the help of Machine Learning, it’s becoming easier and easier. In this article, we’ll be discussing the data and models used in Machine Learning to make predictions about the future.
Data Collection:
To begin with, data collection is a key part of machine learning – it’s essential that you have accurate and comprehensive data on hand before you can begin to make any predictions. For pandemic forecasting, you will need to collect data related to population health, economic activity, government policies, and other relevant factors.
Machine Learning Models:
Once your data is collected, you will need to decide which type of model best fits your situation. There are many different types of machine learning models such as regression models for predicting continuous values or classification models for classifying individuals into distinct categories.
Feature Engineering:
After deciding on a model, feature engineering is essential for training your model. Feature engineering involves selecting important characteristics from your dataset and transforming them into numerical features that can be used as inputs in machine learning algorithms.
Algorithm Selection:
By understanding the strengths and weaknesses of each algorithm available to use in machine learning, you can select an algorithm that will yield the most accurate results with the given dataset. Depending on the situation, some algorithms may be more effective than others when applied to pandemic forecasting tasks.
Hyperparameter Tuning:
Once an algorithm has been selected, hyperparameter tuning involves selecting optimal values for different parameters within a machine learning model so that it performs optimally on unseen data points.
Classification and Clustering Approaches to Predicting Pandemics
Supervised Learning deals with labeled datasets – it seeks to identify patterns in a dataset while labeling data points according to known types or categories. The goal of supervised learning is to predict future events based on past observations – such as infectious diseases outbreaks or pandemic recurrence.
Unsupervised Learning, on the other hand, does not require labels or external categories since all the data are treated equally. This type of Machine Learning technique focuses on finding intrinsic structures within datasets.
Data mining is another powerful tool used in predictive analytics for pandemic forecasting. Data mining techniques involve extracting information from large amounts of data in order to unearth hidden patterns and make predictions about future events or outbreaks.
Utilizing Artificial Intelligence in Pandemic Forecasting
The benefits of using AI in pandemic forecasting are numerous: its ability to sift through massive datasets quickly and accurately can help us gain greater insight into what strategies are more effective and how to better prepare for future outbreaks. AI can also provide invaluable guidance by detecting patterns and uncovering emerging trends which could significantly reduce the time needed for risk assessment, mitigation planning, control strategy implementation, and public health response decision making.
Governments across the world are investing heavily in developing predictive models using machine learning algorithms which make use of complex data sets covering a wide range of parameters including four dimensional weather data, human mobility information, satellite imagery and other remote sensing data.
Using AI in pandemic forecasting allows us to better understand the underlying mechanisms behind infectious diseases while also giving us early warnings about potential outbreaks. This is especially useful during times of crisis like this one – it allows nations to take action before an outbreak reaches its peak, thus reducing its spread significantly.
How to Use Machine Learning for Accurate Predictions
So how do you go about using ML for accurate pandemic forecasting? First, you need to collect valuable data from a variety of sources. This could include disease reports, hospital data, healthcare provider accounts and more.
You can also apply some ML techniques to help you build better models more quickly than traditional methods. For example, if you wanted to predict a potential pandemic outbreak in a given area, your model could benefit from incorporating weather patterns, population density, mobility patterns and more into its prediction strategy.
Predicting pandemics is no easy task — but by using powerful Machine Learning technologies and applying them appropriately to your predictive models, your predictions can become much more reliable and accurate. With the right data collection strategies and machine learning algorithms in place, you can make informed decisions about where and when epidemiological threats may surface – giving yourself a much better chance at staying ahead of any potential outbreaks.
Challenges with Pandemic Forecasting via Machine Learning
The Covid19 pandemic has demonstrated the limits of our ability to forecast its trajectory. While machine learning (ML) has been used for many years in forecasting, doing so for pandemics presents new challenges.
Complexity is also an issue when forecasting using ML models as pandemics involve multiple variables that interact and influence each other in different ways. The unpredictable nature of pandemics makes it even more difficult to develop a reliable ML model that could offer accurate forecasts.
Pandemic forecasting also faces difficulty when trying to accurately model human behavior. Human actions cannot always be predicted due to their ever changing nature which results in unreliable assumptions when it comes time for making predictions.
Benefits of Using Machine Learning for Pandemic Forecasting
One of the primary benefits of using machine learning for pandemic forecasting is improved global health. Machine learning techniques open up new opportunities for analyzing large amounts of data to detect early signs of outbreak and suggest proactive early response strategies.
Another benefit is increased accuracy when it comes to predicting the spread of a disease. With traditional methods, it can be difficult to accurately predict how quickly a disease will spread or where it will become more severe. However, by leveraging machine learning technology, health experts can predict future events more accurately in terms of timing and locations – helping them respond more efficiently with targeted resources in critical areas.
The use of machine learning also helps reduce costs associated with responding to outbreaks. Thanks to sophisticated algorithms that can quickly process large amounts of data, machine learning applications can provide detailed insights about activity patterns that enable officials to make cost effective decisions while still ensuring optimal response times in critical situations.
Implementing machine learning can help improve the accuracy of pandemic forecasting, but it is important to understand the limitations and challenges associated with using it.
However, while machine learning can help improve the accuracy of pandemic forecasting, there are a few key limitations and challenges associated with using it. For example, data collection and cleaning can be time consuming processes. Machine learning models must be trained on datasets that are clean and contain enough relevant information for an ML model to accurately detect trends and patterns in them.
Nevertheless, by utilizing advanced ML algorithms such as deep learning or neural networks, researchers can gain valuable insights into the trajectory of a pandemic which in turn enables them to make informed decisions about future preparedness measures. Moreover, incorporating novel techniques such as natural language processing (NLP) shortly after a pandemic has begun allows for faster prediction speed when compared with traditional methods of forecasting.
All in all, while there are some inherent challenges associated with using machine learning for pandemic forecasting, investing in this technology offers invaluable insights for accurately predicting the future trajectory of any given pandemic.
Comentarios